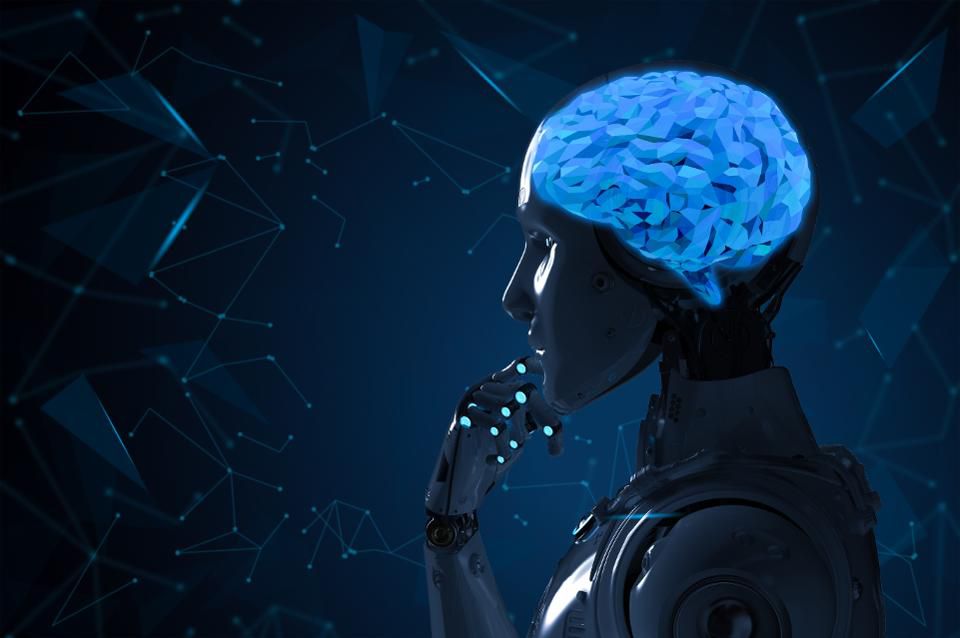
AI Solutions Will Help Businesses Make Key Decisions
November 17, 2020More than half of industry leaders believe that over the next five years, the world will shift the management of high-value assets such as factories, equipment, and machines to AI. This global trend was identified in a study by Siemens and Longitude Research.
More than 500 senior leaders from the energy, manufacturing, infrastructure, and transport sectors took part in the survey regarding the development and implementation of AI.
During the survey, respondents were asked the following questions:
What if you could automate day-to-day operational decisions in your organization so that employees can focus on strategic projects such as developing new product lines or expanding a business? How good does an AI model need to be before you’re ready to hand over control to it? Should its performance be at the level of engineers, or should it surpass it?
In fact, the study showed that the level of trust in AI is already relatively high: 56% of respondents would prefer to implement an ideal AI model instead of looking for an experienced employee (44%). This means that the remaining 44% are likely to have more confidence in decisions made by humans, even if the evidence is in favor of AI.
The study explored the types of contextual data that leaders believe to be the most useful to date. Data from equipment manufactures received the most votes, with 71% rating this as a minor or major benefit. Following this was internal data from other divisions, regions, or departments (70%), supplier data (70%), and performance data from sold products in use with customers (68%).
Utilizing AI in these industries is expected to help avoid accidents and make the workplace safer. 44% of respondents believe that AI-based systems will autonomously monitor machinery that could potentially result in injury or death over the next five years. Even more respondents (54%) believe that AI will autonomously control some of their organization’s high-value assets within the same time frame.
However, in order to transfer this responsibility to AI, industrial AI will need to become more sophisticated. Often this will be driven by new approaches to how data is managed, generated, represented, and shared.
These include, for example, knowledge graphs that reflect the relationship between diverse data sets, and digital twins, allowing you to create detailed digital representations and simulations of real systems, assets, and/or processes. Using industrial knowledge graphs to improve AI models by combining different data sets has clear potential.
To sum up, Norbert Gaus, Head of R&D in Digitalization and Automation at Siemens, stated that “Knowledge graphs add context to the data you’re analyzing. The technical characteristics of a machine can be analyzed in the context of design data, including the task the machine is made for, the temperature at which it must operate, the key parameters, and so forth. Knowledge graphs make it significantly easier to augment the machine data we use to train the AI model, adding valuable contextual information.”
As such, it’s clear that AI will be used to control high-value assets, including machines, equipment, and more which will make both life and work much easier and productive. However, despite the evidence supporting AI-based systems, many people still prefer human labor. Nevertheless, in the nearest future, we can expect and eventually observe the AI recommendations in practice.