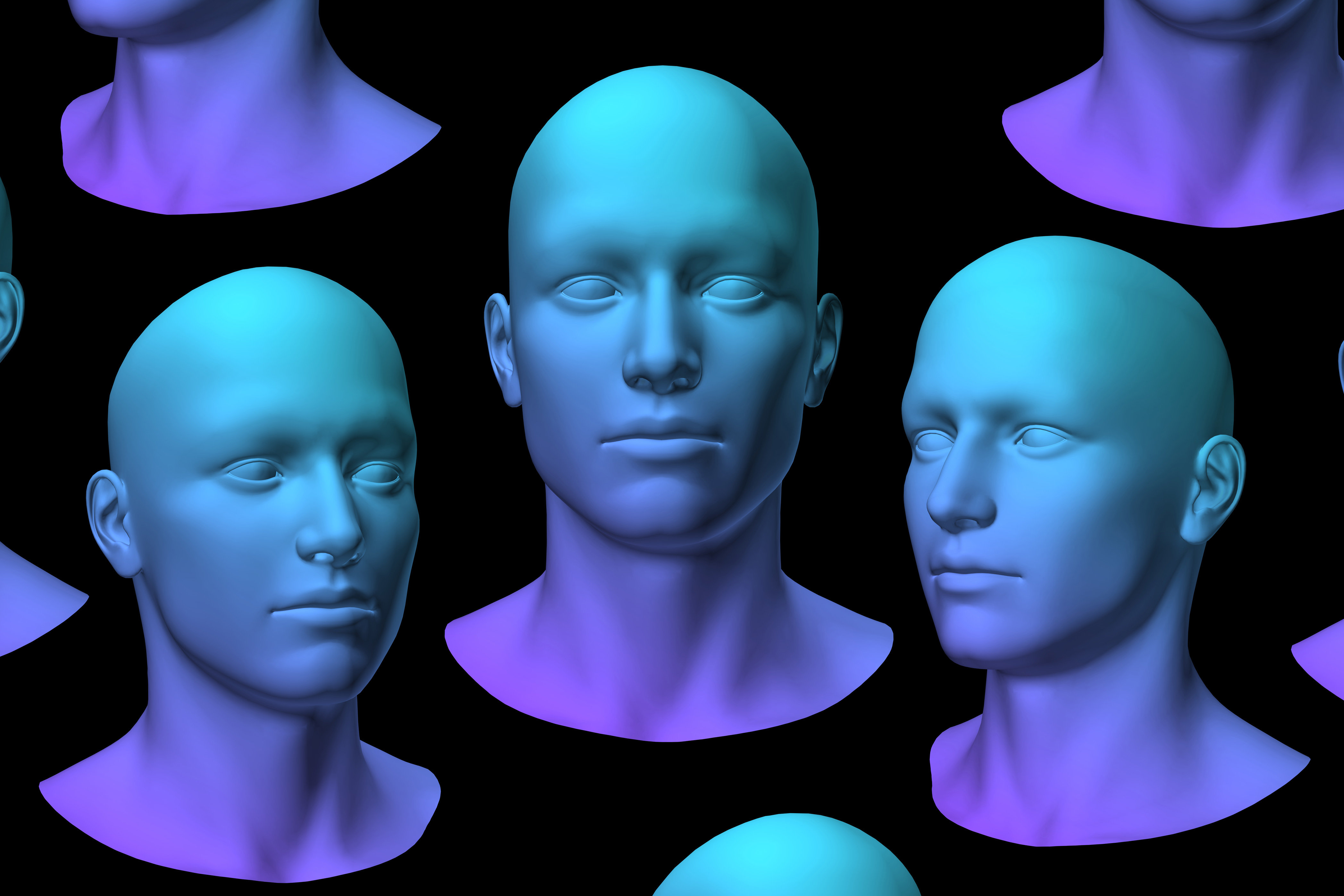
Researchers Taught an Artificial Neural Network how to Generate Individually Tailored “Attractive” and “Unattractive” Faces
March 11, 2021Finnish scientists have developed a generative adversarial neural network that can analyze EEG data and generate artificially produced images of male and female faces, which correspond to an individual's aesthetic preferences.
Testing volunteers in a double-blind procedure found that the accuracy of which the neural network predicted the participants' preferences was over eighty percent.
The study, combining computer science and psychology, involved 30 volunteers comprised of students and staff at the University of Helsinki. The findings have been published in the journal IEEE Transactions on Affective Computing.
Despite being able to easily determine whether a person can be classified as attractive or not, it's relatively difficult to single out which factors we base such a decision on.
Over the years, psychologists have studied this issue. However, they have only been able to highlight general patterns that affect the overall assessment of a person's attractiveness, for instance, the geometry of a face, its symmetry, and the approximately equal ratio of the nose to the forehead and nose to the chin.
Furthermore, experts determined that the criteria for individual preferences are affected by hormones, one's own attractiveness, plus social and cultural experiences. As such, it hadn't been possible to create images that would be consistent with someone's aesthetic preferences.
To overcome this, cognitive neuroscientist Michiel Spape of the University of Helsinki and colleagues first trained a generative neural network to generate artificial portraits from a dataset of 30,000 images of celebrities. Then 240 artificially created images were shown, one at a time, to 30 participants.
The researchers asked the participants to pay attention to the faces they found attractive while their brain responses were recorded via electroencephalography (EEG). The event-related potential (EVP) method was used, which measures the brain's response to a certain sensation, cognitive, or motor event. But before that, the participants were shown eight sets of 4 images and asked to choose the least attractive portrait in each episode.
The EEG data was then transmitted via a brain-computer interface to the generative adversarial network, which was able to interpret the brain's response in terms of how attractive each participant found the images. Using the data, the generative adversarial network was able to generate images specific to each participant.
Two months later, in a second experiment, these artificially generated faces were shown to the participants among other unattractive and neutral images in a matrix of two rows of 12 images. Participants then clicked on those they considered attractive and also rated each image on a scale of 1 to 5, with 1 being "very unattractive" and 5 "very attractive.
Ultimately, the results validated the researchers' study. 86.7% of the tailored-to-be-attractive images were rated as attractive, while only 20% of the images generated to be unattractive were rated as attractive. Furthermore, the neural network generated 20% of false-negative images. Plus, tailored-to-be-attractive images compared to the neutral and unattractive images received higher attractiveness ratings (p <0.0001).
This suggests that the neural network was able to determine to a high degree of accuracy (83.33%) which responses in the brain correlated with viewing an image considered unattractive versus attractive.
At the end of the experiment, the participants were asked how they felt about the study. Participants responded, stating they were satisfied with the results, and some even rated the generated images as examples of "ideal male beauty" and noted the similarity with their partners. A few women were so impressed that they asked for copies of the pictures to keep for themselves.
Considering the success of the study, it's evident that with continued research and development, we should expect to see innovative new research and surprising advancements in neurotechnology.